Epinomy - Recursive Intelligence: AI Workspaces Meet Manufacturing Wisdom
Discover how AI project spaces leverage Deming's PDCA cycle and root cause analysis to transform manufacturing troubleshooting without complex integrations.
Somewhere in a manufacturing plant, a machine fails. Alarms sound. Production halts. Money evaporates with every passing minute. The troubleshooting dance begins—maintenance techs gather, knowledge bases open, and the hunt for root causes commences.
This familiar scenario plays out thousands of times daily across global manufacturing. The response often follows patterns established decades ago through methodologies like the Deming Cycle (Plan-Do-Check-Act) and Five Whys root cause analysis. These approaches have withstood the test of time because they work. They bring structure to chaos, methodically uncovering solutions while preventing recurrence.
Now these venerable methods find themselves transformed—not replaced—by a new cognitive partnership: the AI workspace.
The New Persistence Layer
Modern AI workspaces—Claude Projects, OpenAI's Advanced Data Analysis, Perplexity Spaces, and Anthropic's Claude Sonnet—create something subtly revolutionary: persistent conversation spaces with embedded memory and reasoning capabilities. The innovation isn't the AI itself but the persistence and context.
Traditional chatbots offer isolated interactions without memory. Each question exists in a vacuum, divorced from previous exchanges. These new workspaces preserve context across sessions, maintaining a continuous thread of understanding.
This memory layer transforms troubleshooting from disconnected questions into something resembling human collaboration.
PDCA Reimagined
The Deming Cycle (Plan-Do-Check-Act) has guided manufacturing excellence for generations. Originally conceived by Walter Shewhart and popularized by W. Edwards Deming, this iterative approach provides structure for continuous improvement. Let's examine how AI workspaces enhance each phase:
Plan: Enhanced by Collective Knowledge
The planning phase traditionally begins with problem definition and data collection. AI workspaces supercharge this through what amounts to assisted institutional memory.
A maintenance technician investigating a failed motor can prompt the workspace with equipment details, error codes, and observed symptoms. The AI then:
- Retrieves relevant facts from its training data spanning millions of engineering documents
- Connects symptoms to potential causes based on similar historical failures
- Organizes data into structured hypotheses for testing
- Suggests testing procedures and necessary tools
- Creates a clear troubleshooting roadmap
What's striking isn't that the AI provides answers—it's that the entire planning conversation remains accessible throughout the process. Details aren't lost in shift handovers or forgotten notes. The workspace becomes a living document of the investigation.
Do: Documentation in Real Time
During implementation, technicians traditionally record their actions and observations in logs or maintenance systems. AI workspaces provide a conversational interface for this documentation:
"I've checked the capacitor values and they're within spec, but I'm seeing unusual harmonics on the oscilloscope."
These observations remain in context, allowing the AI to immediately compare results against expectations and suggest adjustments. More importantly, this seamless documentation happens naturally through conversation rather than through separate form-filling exercises, dramatically increasing compliance.
Check: Analysis Through Dialogue
The verification phase benefits perhaps most dramatically from AI workspaces. Results can be discussed conversationally:
"The motor's now running, but I'm still seeing elevated temperatures on bearing assembly B."
The AI can help analyze whether the intervention succeeded, partially succeeded, or failed. It can suggest additional measurements or tests to verify root causes have been addressed, flagging potential complications or secondary issues that might emerge.
This dialogue-based analysis mimics consultation with experienced colleagues, drawing out details through follow-up questions while maintaining the broader context of the investigation.
Act: Standardization and Knowledge Transfer
The final phase—implementing successful solutions permanently or beginning a new cycle—transforms through AI workspaces' ability to synthesize information. The system can:
- Draft formal documentation of the resolution
- Create standardized procedures for similar future issues
- Identify preventive maintenance modifications
- Generate training materials for other technicians
Most valuably, this knowledge remains accessible for future troubleshooting sessions. Six months later, when similar symptoms appear, the entire historical thread can be referenced instantly.
Root Cause Analysis Without Root Pain
Manufacturing's embrace of techniques like Five Whys analysis provides structured approaches to finding underlying causes rather than treating symptoms. AI workspaces bring fresh capabilities to this process:
Hypothesis Generation at Scale
When faced with machinery failure, AI systems can rapidly generate multiple potential root causes based on symptoms, creating a comprehensive investigation map. Unlike human experts who might fixate on favorite theories, AI workspaces easily maintain multiple parallel hypotheses.
A technician investigating a production line stoppage might receive:
"Based on the symptoms, we should investigate these five potential root causes: 1. Power supply voltage fluctuations 2. Worn encoder bearings 3. Control system timing errors 4. Network communication latency 5. Material feed inconsistencies"
This structured approach helps avoid premature closure—the tendency to stop investigating after finding the first plausible explanation.
The Fifth Why and Beyond
The Five Whys technique—asking "why" recursively to drill down to root causes—transforms in AI workspaces through structured dialogue. The system can maintain the questioning thread without the facilitator bias that sometimes emerges in human-led sessions.
When analyzing a product quality issue, the conversation might flow:
Human: "We're seeing surface finish defects on the machined parts."
AI: "Why are surface finish defects appearing?"
Human: "The cutting tool is wearing prematurely."
AI: "Why is the cutting tool wearing prematurely?"
Human: "The coolant concentration is inconsistent."
The AI maintains this structured inquiry while simultaneously building a causal diagram of the emerging understanding. When the investigation completes, it can generate a comprehensive root cause tree showing the relationships between factors—something that typically requires separate documentation steps.
The Power of Focus
Perhaps the most underappreciated aspect of AI workspaces for manufacturing lies not in what they add but in what they eliminate: context switching.
Manufacturing troubleshooting traditionally involves a cognitive juggling act: - Reading equipment manuals - Searching knowledge bases - Consulting with colleagues - Documenting findings - Switching between systems
Each context switch imposes a cognitive tax, fragmenting attention and increasing error rates. AI workspaces consolidate these activities into a single interface, reducing the mental overhead and allowing deeper focus on the actual problem.
A maintenance technician can maintain a single conversation thread that includes equipment specifications, historical failure data, testing procedures, and real-time analysis—all without switching applications or hunting through disconnected resources.
Before the APIs, After the Revolution
What makes this transformation remarkable is that these capabilities exist before any formal system integration. Even without API connections to maintenance systems, historians, or control networks, AI workspaces already provide significant value through their contextual understanding and persistence.
When these integrations inevitably arrive, we'll see further amplification as AI workspaces gain direct access to real-time telemetry, maintenance histories, and control systems. But the cognitive partnership model is already transforming troubleshooting practices.
The New Manufacturing Extension
Manufacturing 5.0—the emerging vision of human-machine collaboration—finds a perfect expression in AI workspaces. Unlike automation that replaces human judgment, these systems amplify human capabilities while preserving the irreplaceable values of experience and intuition.
The AI doesn't dictate solutions; it organizes information, maintains context, suggests possibilities, and documents understanding. The human remains central—making decisions, performing physical interventions, applying judgment, and feeding observations back into the system.
This partnership model preserves the best aspects of manufacturing culture: the focus on systematic improvement, the value of experience, and the continuous learning cycle. It simply accelerates these processes while reducing their cognitive burden.
Ripples Beyond the Factory Floor
The manufacturing model of structured problem-solving spreads easily to other domains when enabled by AI workspaces. Supply chain disruptions, customer service challenges, and software development bugs all benefit from the same systematic approach.
The crucial insight lies in recognizing that these AI workspaces aren't merely chat interfaces—they're cognitive partners that maintain shared context across time. They represent perhaps the most significant advancement in collaborative work since the spreadsheet, though in a form that feels deceptively familiar.
What manufacturing discovered through decades of quality methodology development—the value of structured inquiry, methodical testing, and systematic improvement—now becomes accessible to broader audiences through conversational interfaces that guide without constraining.
The best manufacturing plants have always been learning organizations. Now that learning happens in partnership with systems that never forget, never tire, and continuously improve. The dance between human expertise and machine memory has only just begun.
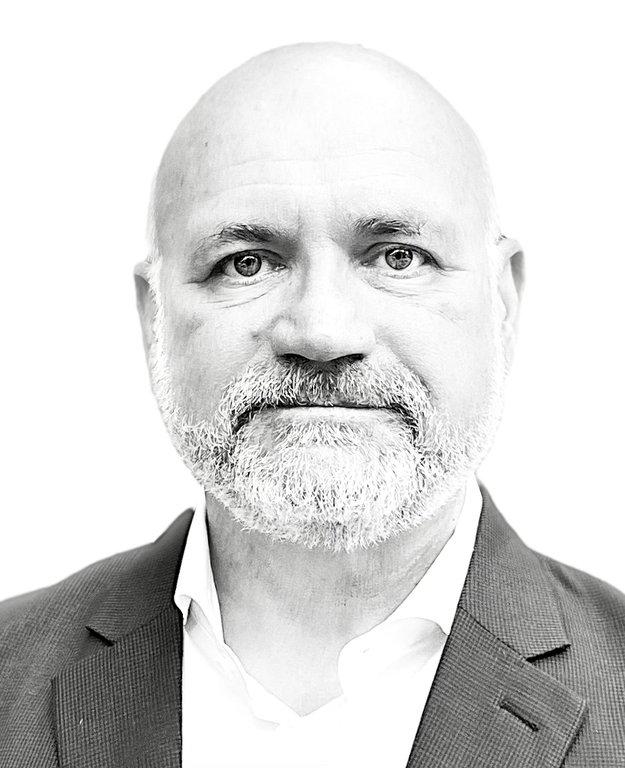
Geordie
Known simply as Geordie (or George, depending on when your paths crossed)—a mononym meaning "man of the earth"—he brings three decades of experience implementing enterprise knowledge systems for organizations from Coca-Cola to the United Nations. His expertise in semantic search and machine learning has evolved alongside computing itself, from command-line interfaces to conversational AI. As founder of Applied Relevance, he helps organizations navigate the increasingly blurred boundary between human and machine cognition, writing to clarify his own thinking and, perhaps, yours as well.
No comments yet. Login to start a new discussion Start a new discussion